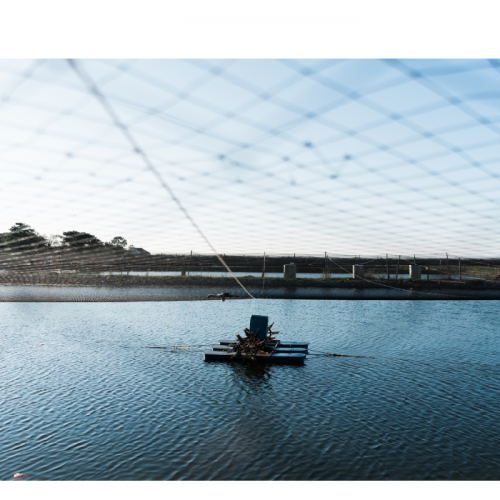
Optimisation of Feeding Strategies at A Fish Farm Through Mathematical Modelling
| Wed, 18 Nov 2020 - 13:24
Optimisation is a keyword that bounces inside every fish farmer's head. Nowadays, more than ever, fish farmers seek to optimise every single aspect of their businesses, a motivation that is imposed by the increased competitiveness within the fish farming industry. This pressure is particularly felt in the EU, where aquaculture production has been stagnant in terms of volume (FAO, 2020) and generally subjected to global fish price reductions (e.g., seabream and seabass).
This means that, in order to keep businesses running with attractive profits, fish farmers are continuously restructuring their operations, seeking optimisation and efficient production. Looking into the different manageable resources and operations of a fish farm, feeding is usually the one process that represents the greatest weight in the cash outflow, accounting for 30-60 percent of total operational costs. Thus, feeding is one of the main resources and operations that have been targeted for optimisation.
In the past few years, the aquaculture industry has seen significant advances with regard to feeding optimisation. These advances have been made at multiple levels, covering areas that span from nutrition to operation monitoring, e.g., feeds formulated based on more precise nutritional requirements and use of underwater cameras to assist in feeding operations. More recently, this industry has seen the emergence of decision-support tools that leverage the potential of mathematical algorithms. Examples include tools based on mechanistic mathematical models that describe fish growth, feed conversion and waste production, therefore showing great potential to support feeding optimisation activities. Although these types of tools have been used by the scientific community since the 90's (e.g., Conceição et al., 1998; Lupatsch and Kissil, 1998; Nobre et al., 2019), only recently did they become available to be used by non-modelling experts and generalised for fish farmers.
Also read: Maine Engineer Develops Low-cost Monitoring Buoy
In this article, we illustrate an application of a mechanistic nutrient-based model to optimise the feeding performance in a pre-growing unit of a commercial farm (Piscicultura Vale da Lama, Portugal). For that purpose, we have used the FeedneticsTM model in a typical two-step approach:
Gaining confidence in model robustness, where the FeedneticsTM model performance was evaluated by comparing the model predictions against historical data of European seabass grown in the pre-growing unit;
Assessing the performance of different feeding strategies, where the performance of European seabass fed under different feeding strategies was compared in terms of growth, feed conversion, economic conversion, total N waste and total solids waste.
Gaining confidence in model robustness
FeedneticsTM is a web-application that includes a mechanistic nutrient-based model which predicts fish growth and composition along time. This web-application uses information on temperature, feed intake and feed properties.The model has been calibrated with a large and diverse range of datasets and is currently available for gilthead seabream, European seabass, and rainbow trout.
Also read: Scientists Accidentally Create New Fish
Aiming at demonstrating model robustness, in the first step of this work, the predictions of the FeedneticsTM model were evaluated against historical data of Piscicultura Vale da Lama, a commercial fish farm located in Portugal. The historical data set includes information about six pre-growing tanks of European seabass and contains daily resolution information, part of which was recorded automatically by sensors and partially manually by the technical staff throughout the production period (i.e., temperature, number of fish, amount and type of feed). Data regarding feed properties was based on information shared by the commercial farm, which was mainly taken from the official technical sheets and feed labels provided by the respective feed producers. For the cases where there was a lack of essential data to run the model (e.g., digestibility coefficients and amino acid profiles), default values that comply with the nutritional requirements of the species under evaluation were assumed.
Figure 2 shows a comparison between predicted and measured body weight values. Overall, the FeedneticsTM model accurately predicts the body weight for this historical seabass data set, presenting a mean absolute percentage error (MAPE) of about 8.9 percent. Given these positive results, we can say that the model can be used with confidence to assess the performance of different seabass feeding strategies at this commercial farm.
Assessing the performance of different feeding strategies
Getting clear conclusions about the performance of different feeding strategies based on the direct analysis of historical data can be tricky. Among others, the main difficulties are associated to the fact that it is not straightforward to separate the effects related to nutrition from those related to other factors (e.g., temperature). Decision-support tools based on mathematical models can be a major asset in this regard, since they allow to quickly compare different feeding strategies while keeping other unrelated factors fixed.
In the second step of this work, FeedneticsTM was used to assess the performance of European seabass when fed under three different feeding strategies (A, B and C), during the pre-growing phase of the Piscicultura Vale da Lama farm. All feeding strategies share a common plan, only differing in terms of the commercial feeds provided during the time periods of 34-54 days and 66-135 days. The amount of feed provided in each scenario was managed based on the Piscicultura Vale da Lama's feeding table, which is adjusted for its specific farming and environmental conditions.
Similar to what was reported in the previous section, the majority of the data regarding the feed properties was based on information available in the official technical sheets and feed labels of the respective feed producers. For the cases where there was a lack of essential data to run the model (e.g., digestibility coefficients and amino acid profiles), default values that comply with the nutritional requirements of European seabass were assumed. Table 1 shows the characteristics of the three different commercial feeds (A, B and C) under evaluation. The characteristics of the common commercial feeds are not shown, since they are transversal to all feeding strategies.
Also read: Fish Back On The Menu with Oxair’s Yield Boosting Technology
The three different feeding strategies were run in FeedneticsTM for a production period of 137 days, considering an initial average body weight of 18.4g, an initial number of fish of 57,000 individuals, a mortality rate of about 4.7 percent and a historical temperature profile (13.9-25.3 °C). For the farming and environmental conditions considered, the model predictions suggest that following feeding strategy B (FBW = 117.2 g) leads to a higher growth compared to feeding strategies A and C (FBW = 104.9 and 106.6 g, respectively). In terms of feed and economic conversion, feeding strategy B also presents the best results (FCR = 1.07 and ECR = 1.51 Eur/kg) and, therefore, is the most cost-efficient one for the farming and environmental conditions of Piscicultura Vale da Lama pre-growing unit, allowing savings on feed of about EU €290-670-per-tonne of fish produced, compared to feeding strategies A and C respectively.
In addition, the best performance of feeding strategy B is consequently translated in terms of waste production, resulting in lower waste outputs of around 13 percent and 12 percent (total N waste and total solids waste, respectively), in comparison to feeding strategies A and C. Ultimately, this lower waste production, that is obtained by following feeding strategy B, can contribute to maintaining better water quality and minimising environmental impact.
Final remarks
Decision support tools that leverage the potential of mathematical models can be important assets for fish farmers, supporting the constant seeking for a more efficient production system. By allowing the comparison of multiple feeding scenarios, while keeping factors unrelated to nutrition fixed, these types of tools provide a strong basis for feeding evaluation and optimisation. This is something that may be difficult to achieve through historical data analysis alone, especially at a time when feed producers are commercialising aquafeeds which incorporate increasingly higher levels of sustainable ingredients.
This article illustrates how mathematical models, like FeedneticsTM, can be used to optimise feeding strategies from a cost-efficiency perspective. Moreover, models with this high level of detail (e.g., considering amino and fatty acids profiles of feed and fish) can also be used to optimise feeding strategies from a value-added perspective. For instance, different feeding strategies can be evaluated using these types of models in order to produce fish with higher omega-3 fatty acids content at more attractive costs.
Source: aquafeed.co.uk